The difference between capturing a sale and losing a sale often comes down to milliseconds. Retailers need agile, data-driven engagement strategies to capitalize on every opportunity for conversion and upselling. However, the challenge lies in continuously optimizing the shopping experience without relying on rigid, time-consuming testing methods.
Traditionally, A/B testing has been the go-to method for testing different variations of search and recommendation models. While effective, it lacks real-time adaptability and dynamic traffic distribution, two critical factors in today’s competitive ecommerce landscape. This is where self-learning personalization models, powered by the Multi-Armed Bandit (MAB) approach, come into play.
How does Multi-Armed Bandit differ from conventional A/B testing?
As an ecommerce retailer evaluating different recommendation models—such as Diversity, Newness, and 1:1 Personalization—you would want to determine which model best aligns with your current business objectives.
In the standard A/B testing environment, each model receives a fixed share of traffic, with results declared only after a testing period, which can span weeks. During this time, your traffic may be wasted on a suboptimal model— leading to lost opportunities.
However, with the MAB approach, while the initial setup remains unchanged, the system consistently learns from user interactions as they occur, dynamically reallocating traffic to the highest-performing model.
This swift and efficient process ensures that if the newness model is driving the most engagement and conversions, MAB quickly allocates traffic towards it, reducing exposure to underperforming models.
This dynamic adaptation is what sets MAB apart from traditional A/B testing. But how does it achieve this level of efficiency?
The answer lies in its ability to segment traffic intelligently, balance exploration with exploitation, and adapt in real-time—ensuring that each user experiences the most relevant recommendations. As an intuitive site search and product recommendation engine, Netcore Unbxd leverages MAB to drive recommendations at a granular level. Let’s break down how MAB optimizes traffic distribution for personalized recommendations.
The role of Multi-Armed Bandit in personalized recommendation
Here’s how MAB fine-tunes recommendations for each shopper:
Adaptive traffic distribution:
-
The MAB approach segments traffic based on user characteristics such as device type (mobile, desktop), visit type (new, returning), and region (e.g., New York, London, Tokyo). This 'adaptive traffic distribution' means each segment receives the most relevant recommendations, enhancing user experience and increasing the likelihood of conversion.
-
Each segment is assigned an initial equal distribution of traffic across multiple recommendation models.
Exploration and exploitation:
-
Initially, the MAB approach explores all models equally, gathering data on their performance.
-
It also identifies the models generating the highest Click-Through (CTR), conversions, or engagement as data accumulates.
-
MAB then exploits the best models, dynamically assigning more traffic to top performers while phasing out ineffective ones.
Quick optimization:
-
MAB continuously monitors performance and redistributes traffic on the fly.
-
Unlike static A/B testing, which requires waiting until an experiment concludes, this approach ensures that users always experience the best-performing personalization in real-time.
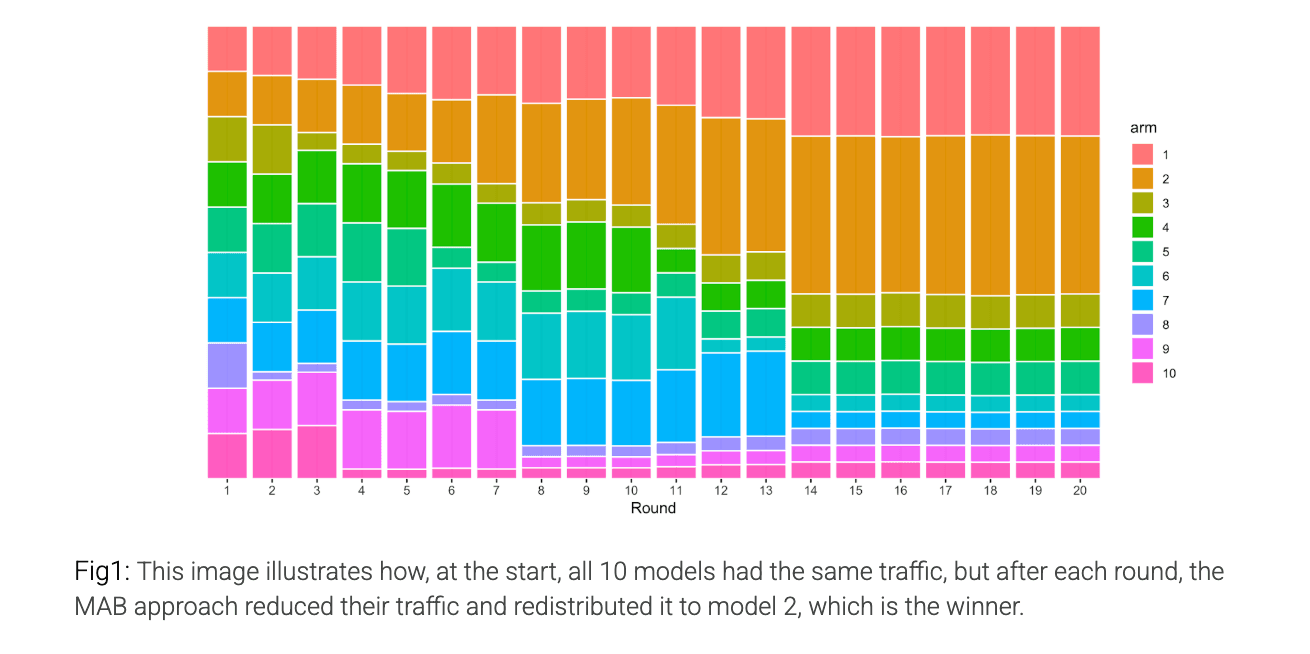
Now that we’ve explored how MAB dynamically optimizes traffic distribution, understanding the key differences between MAB and A/B testing approaches can help marketers and ecommerce leaders make more informed decisions about their experimentation strategies.
Multi-Armed Bandit (MAB) approach vs A/B testing
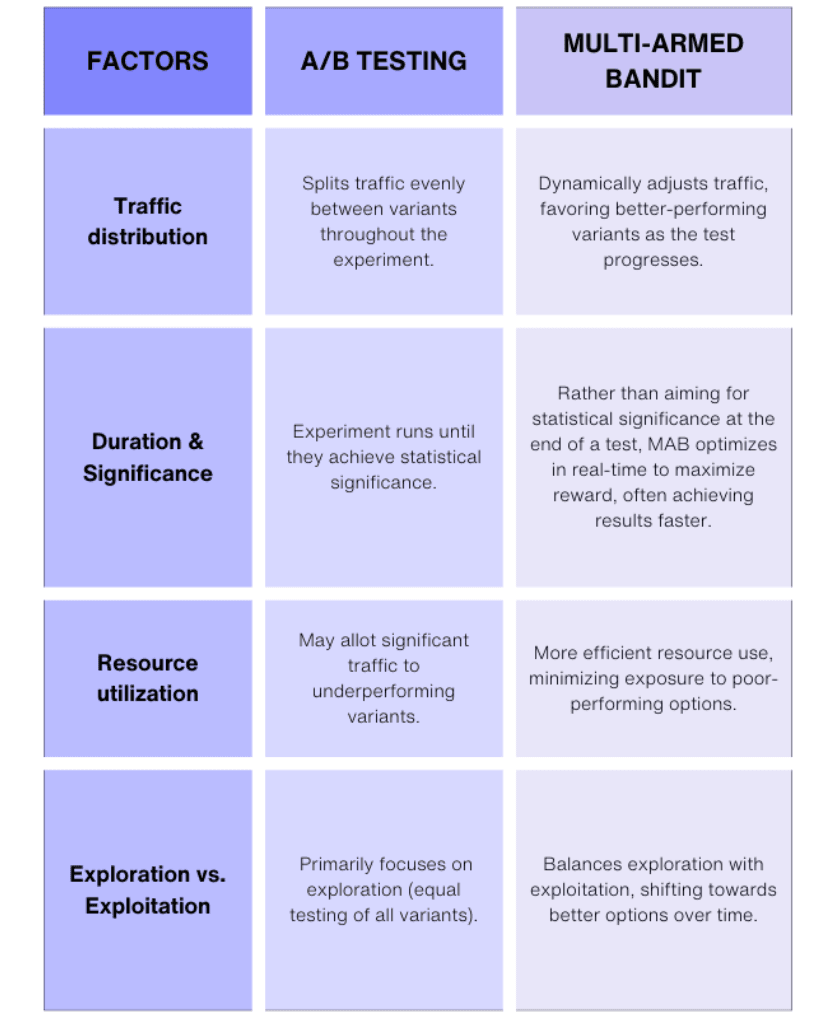
The importance of Multi-Armed Bandit for ecommerce retailers
Across retail verticals, MAB provides several competitive advantages:
1. Efficiency over traditional A/B testing
MAB testing takes a different approach from traditional A/B testing by using machine learning to determine which variants of a personalization model are more effective.
Therefore, instead of running tests and waiting for results, MAB testing constantly analyzes data in real time.
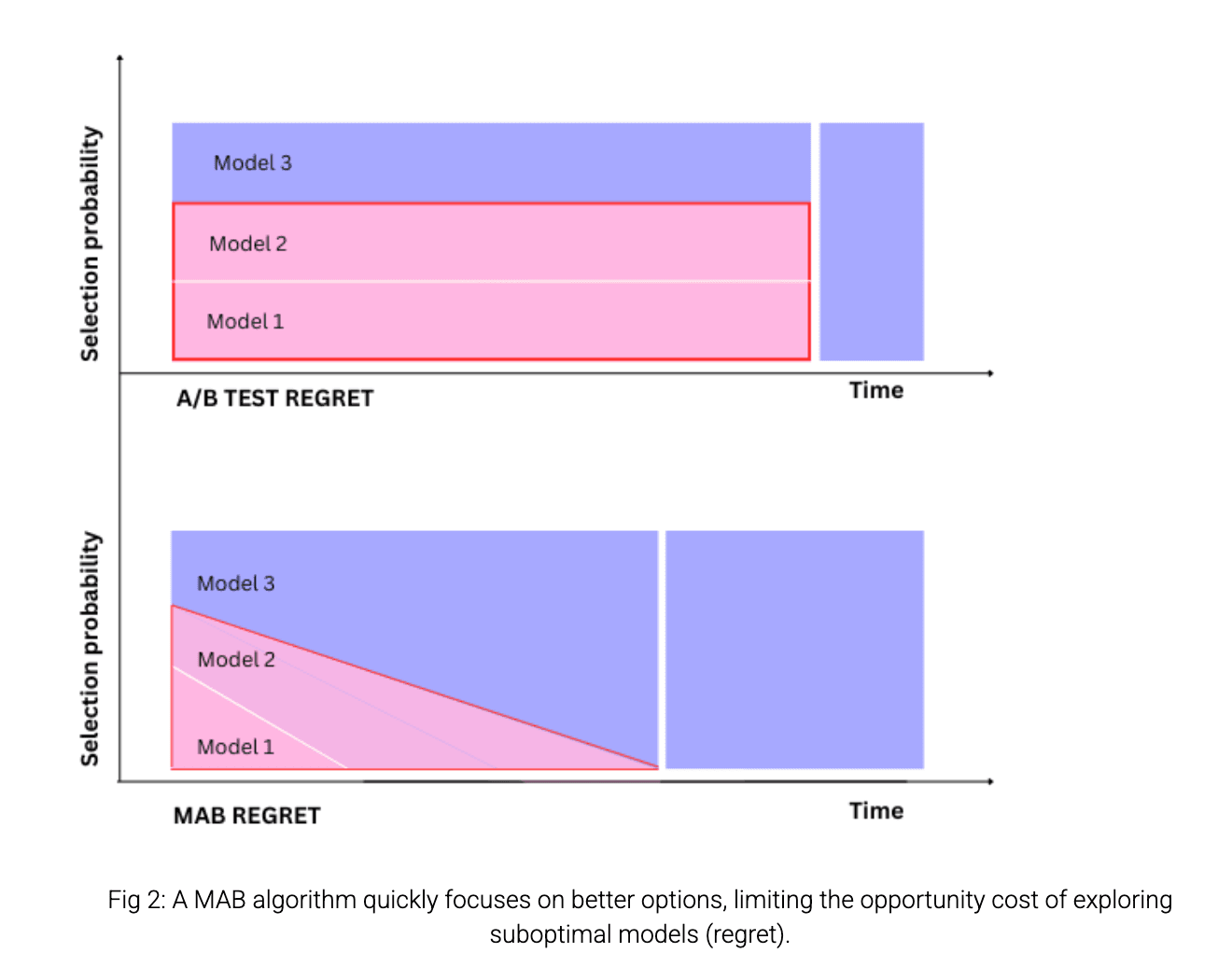
2. Personalized shopping journeys
Customers have different behaviors, preferences, and intents. MAB optimizes experiences for each segment, ensuring high engagement and satisfaction.
This personalized approach makes your customers feel understood and catered to, enhancing their shopping experience.
3. Continuous learning and adaptation
Unlike A/B tests that end once a winner is declared, MAB continuously refines traffic distribution, adapting to seasonal trends, changing user behaviors, and new inventory.
Takeaway
With Netcore Unbxd’s MAB approach, retailers can go beyond static testing and create truly dynamic shopping experiences that evolve with user preferences.
Allocating traffic to high-performing models can help ecommerce businesses maximize engagement, boost conversions, and create highly relevant shopping experiences. To improve product discovery and increase sales, explore our MAB-powered personalization.
To see it in action, schedule a demo now.